A scientific look at adaptive learning paths in EVOLUTION³.
Digitalisation is fundamentally changing learning. Standardised learning paths are increasingly giving way to personalised and dynamic learning processes that adapt flexibly to the knowledge level, needs and pace of learners. EVOLUTION³ offers adaptive learning paths to create an individualised and motivating learning environment.
A decisive factor in the success of adaptive learning systems is the design of the learning processes. The didactics of adaptive learning clearly defines goals, content and target groups and focuses on the individual needs and progress of learners. Modern methods such as simulations and interactive games help to convey content in a clear and practical way. The choice of the appropriate learning format – synchronous or asynchronous, face-to-face or virtual – and interactive design have a decisive influence on learning success.
The selection and sequencing of learning content also plays a key role. The adaptive structure determines the learning path based on real-time data and user preferences. This ensures that the content is optimally tailored to the respective skill level and that learners are neither under- nor overchallenged.
Adaptive learning is just one method of knowledge transfer that requires decisions to be made at various points. The choice between linear, exploratory or adaptive learning structures depends on the learning objectives and the target group. Organisations must decide to what extent adaptivity makes sense at the individual learner level, the content level or for entire learning paths. Depending on the context, combining different methods can ensure the greatest learning success.
But what prerequisites are necessary, what opportunities and challenges arise, and how can artificial intelligence further advance this development?
Didactics
- Learning objectives: Knowledge, ability, understanding, sensitisation
- Learning content: Information, knowledge, instructions
- Target groups: Specialist, demographic, regional
Methodology:
- Form of learning: Presence, virtual, synchronous, asynchronous
- Methods: Presentation, interaction, simulation, game
- Structure and sequence: Linear, explorativ, adaptiv
- Posture: learner-centred, teacher-centred
- Degree of interactivity
- Appearance and tonality
What is adaptive learning?
Adaptive learning is an innovative concept that uses digital technologies to customise learning content to the user’s level of knowledge, skills and learning preferences. In contrast to traditional, linear learning structures that prescribe a fixed sequence, adaptive learning adapts dynamically and in real time to individual learning progress. This personalisation leads to more efficient knowledge transfer, as content is provided exactly when it is needed.
However, implementation requires strategic planning. Companies and educational institutions need to define clear goals, whether it is to improve learning success, optimise learning time or specifically promote specialist skills. In addition to an efficient infrastructure, didactic and technological expertise is essential in order to effectively design adaptive mechanisms. Advanced analysis tools and learning analytics play a central role here. They enable a detailed evaluation of learning behaviour and help to identify strengths and weaknesses. At the same time, data protection and company regulations must be taken into account in order to ensure the secure and ethically acceptable use of learning data. Ultimately, adaptive learning not only opens up new ways of imparting knowledge, but also promotes sustainable, self-determined learning and improves the learning experience in the long term.
Basics of learning design
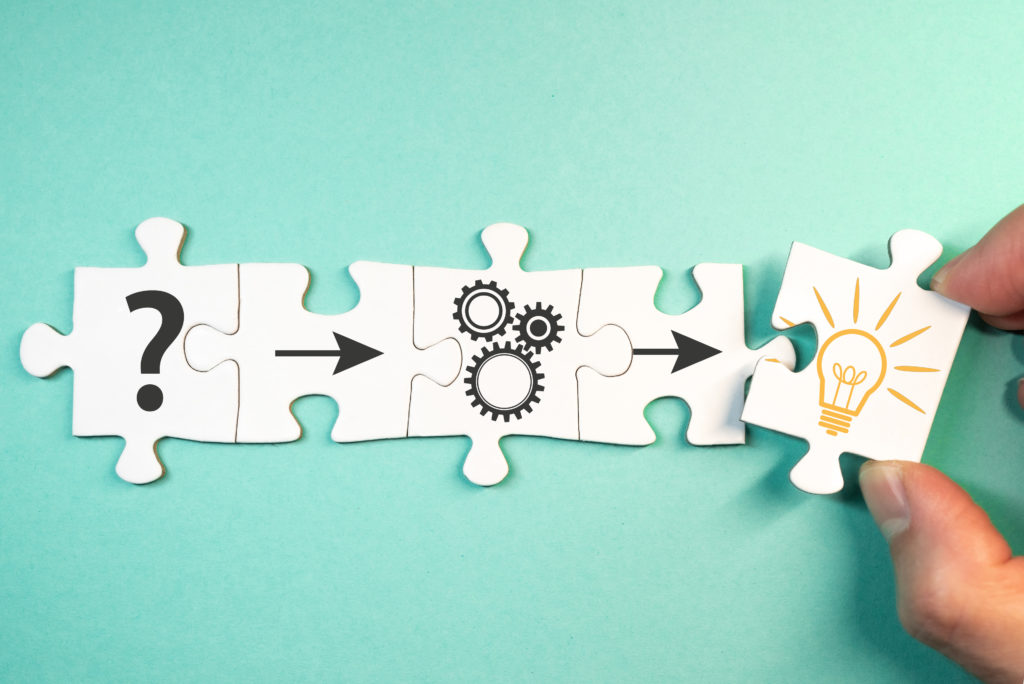
The basis for adaptive learning is a sound didactic concept that takes different learning scenarios into account. Learning content must have a modular and granular structure to allow for flexible customisation. For effective learning design, it is crucial to consider not only cognitive aspects, but also metacognitive and affective aspects, as these significantly influence learners’ motivation and success when engaging with content. Adaptivity can only be implemented in a meaningful way if the structure of the learning material is precisely analysed and broken down into meaningful learning units.
A central principle in adaptive learning is mastery learning. According to this principle, learners only move on to the next learning unit once they have demonstrated that they have understood the content. This is based on continuous, data-supported diagnostics that accurately record the individual learning status. By implementing a formative assessment process, automated analyses and evaluations based on learning paths, it is possible to determine in a targeted manner which content has already been mastered and where repetition or consolidation is necessary. These diagnostic procedures are crucial for personalising the learning process, identifying knowledge gaps at an early stage and ensuring long-term learning success.
Forms of adaptivity
Adaptive learning can take various forms. Content adaptivity adapts the learning material to the individual level of knowledge, methodological adaptivity combines different teaching methods depending on the learning style. Sequential adaptivity optimises the order of content according to learning progress, while social adaptivity is supported by interactions with tutors or other learners. These forms can be combined to provide a seamless and effective learning experience.
One particularly innovative approach is predictive learning, in which algorithms predict future learning needs based on user behaviour and create personalised learning suggestions. In combination with learning analytics, this creates a data-driven ecosystem that continuously analyses and optimises the learning progress of learners.
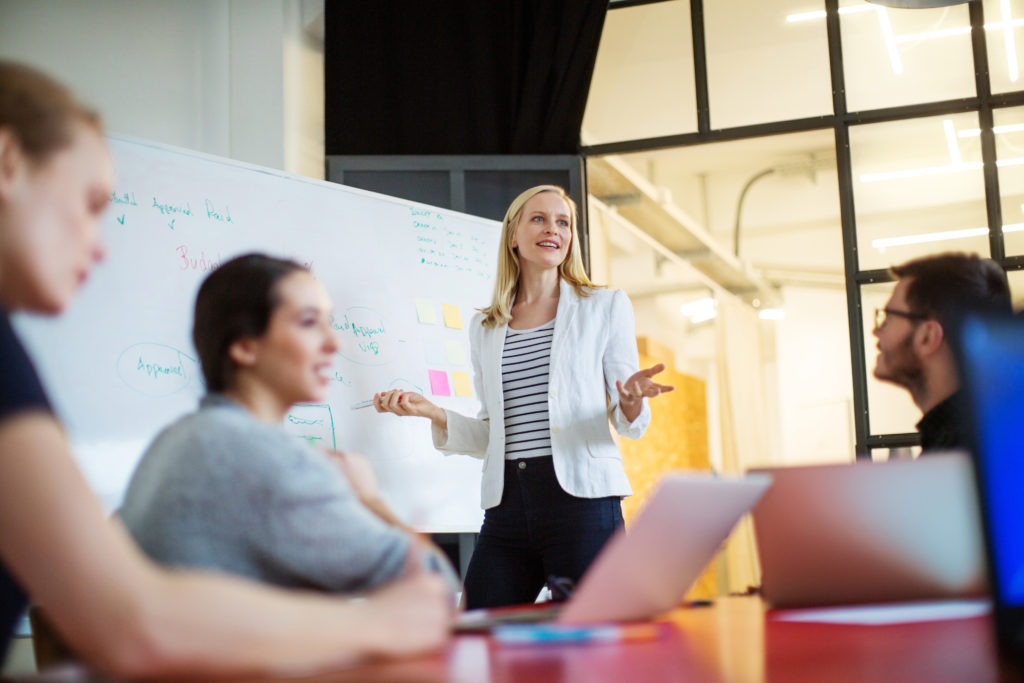
Unlocking potential through adaptive learning concepts
Discover EVOLUTION³ and experience personalised knowledge transfer. Dynamic adaptation keeps your participants motivated and helps them achieve their goals faster. Get started now and take your educational offering to the next level!
Requirements for adaptive learning
The successful implementation of adaptive learning paths requires both conceptual and technological prerequisites. Adaptive learning functions are often integrated into LMS or LXP environments in which closed or modular units form an individual learning path. Adaptivity is based on knowledge level, learner type or role and enables a customised learning experience through a dynamic selection of content. The prerequisites for successful adaptive learning are a stable field of knowledge, clearly defined learning objectives and a sufficient amount of content.
A sound understanding of the learning material and an efficient technical infrastructure are essential in order to optimise the use of adaptive mechanisms. At the same time, detailed analyses and comprehensive reporting play a crucial role in identifying learners’ strengths and weaknesses and adapting content accordingly. This not only maximises learning success and optimises learning time, but also increases the relevance of the content. The combination of personalised learning and technological development is shaping the future of didactics in the long term.
Conceptual and content-related requirements
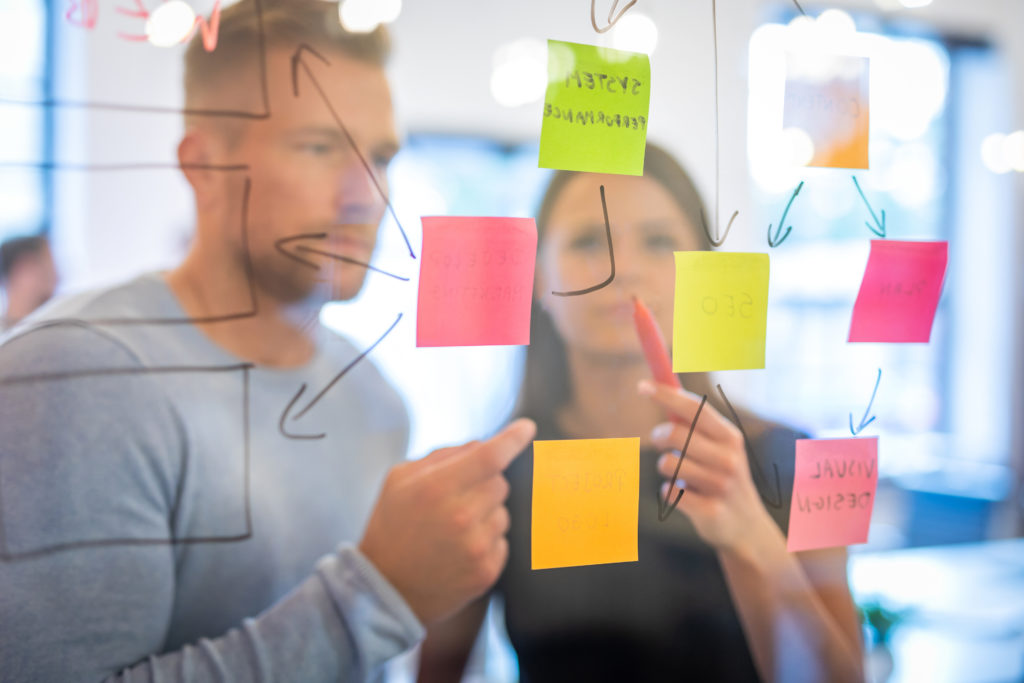
For adaptive learning systems to function efficiently, the learning objectives must be clearly defined and the content carefully structured. Knowledge domains with stable and verifiable content are particularly suitable, as adaptive algorithms can make targeted differentiations here. Depending on the application, different learning structures are used or can be combined with each other. Linear learning paths follow a fixed sequence, explorative learning structures allow the learner to navigate through the content independently, while adaptive structures adapt the path to the individual learning progress.
In addition, role-specific and knowledge-based learning paths play a central role. While role-specific learning paths adapt content to the professional or functional role of the user, knowledge-based learning paths are based on existing knowledge and identified learning needs. Both approaches require sound content and didactic expertise in order to manage learning processes effectively and avoid misalignments. By combining flexible structures and intelligent adjustments, learning motivation can be increased and knowledge acquisition made more sustainable.
Another important concept is Competency-Based Learning (CBL). Here, competences are used as a benchmark for learning progress instead of fixed learning times. This allows for greater individualisation and ensures that learners have demonstrably developed their skills before moving on to more advanced content.
Technical requirements
System-side adaptivity in the LMS/LXP enables the centralised control and management of learning paths that dynamically adapt to the learning progress and preferences of the learners. This facilitates scalability and standardised tracking, but requires high quality and structuring of the stored content. As an alternative to central control via a learning management system (LMS), adaptivity can also be integrated directly into the learning content. In this approach, diagnostic elements are embedded in the learning module. These are used on the basis of user interactions, input patterns or response times. This makes it possible to apply adaptive decision-making logic. This approach is particularly suitable for simulation-based, interactive or scenario-driven formats, as it allows real-time responses to individual learning progress and dynamic adaptation of the content. In this case, AI-supported or workflow-based algorithms take over the fine-tuning of the content to ensure immediate adaptation to the learner. Both approaches require a powerful infrastructure and a well thought-out didactic design to enable an optimised adaptive learning experience.
On a technical level, powerful learning platforms with intelligent data processing are required. Learning Management Systems (LMS) and Learning Experience Platforms (LXP) must not only support metadata-based content organisation, adaptive sequencing and differentiated reporting, but also offer flexible interfaces for exchange with external systems. A key decision is whether adaptivity takes place primarily in the LMS/LXP or in the learning content itself.
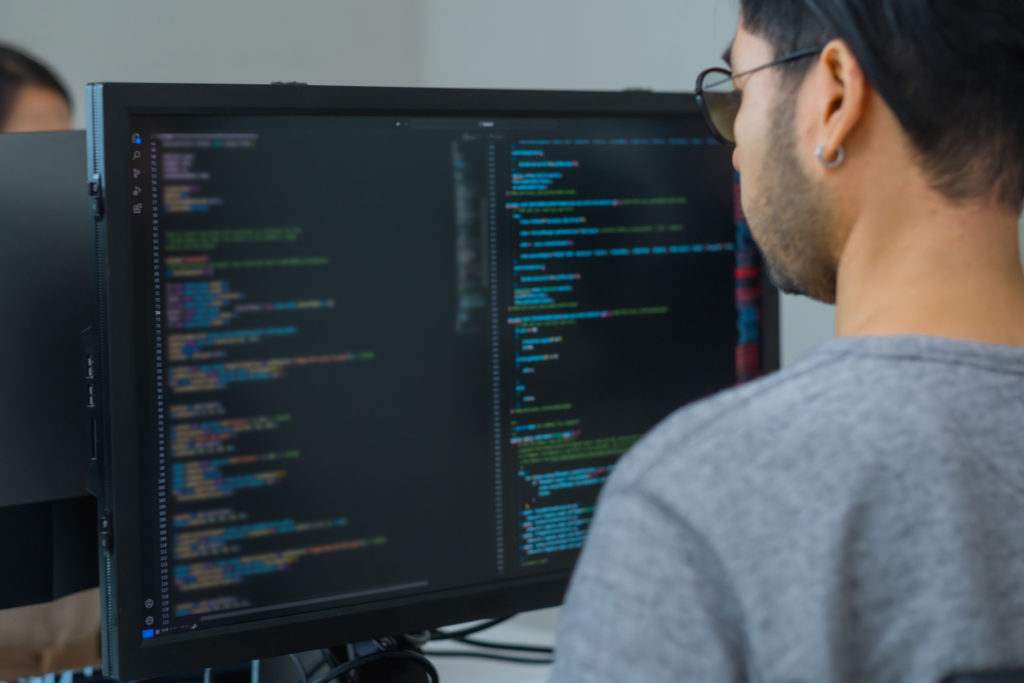
Interoperability between different systems is a particular challenge. Standards such as xAPI (Experience API) enable detailed recording and analysis of learning activities that go beyond traditional LMSs and can map a broader spectrum of learning processes.
Advantages and challenges of adaptive learning
Adaptive learning is a future-oriented approach in which learning processes are specifically tailored to the individual needs and prior knowledge of learners. Data-driven technologies are used to create personalised learning paths that improve learning outcomes and increase participants’ motivation and engagement. Companies and educational institutions benefit from flexible training programmes, optimised skills development and the ability to analyse and manage learning progress in real time.
However, implementation places high demands on strategy, technology and content production. Learning content must be modular, up-to-date and structured in a didactically meaningful way to enable adaptive control. At the same time, legal and organisational framework conditions, in particular data protection, must be taken into account from the outset. Sound planning is the basis for adaptive learning, which offers the opportunity to impart knowledge in a more sustainable, targeted and effective manner.
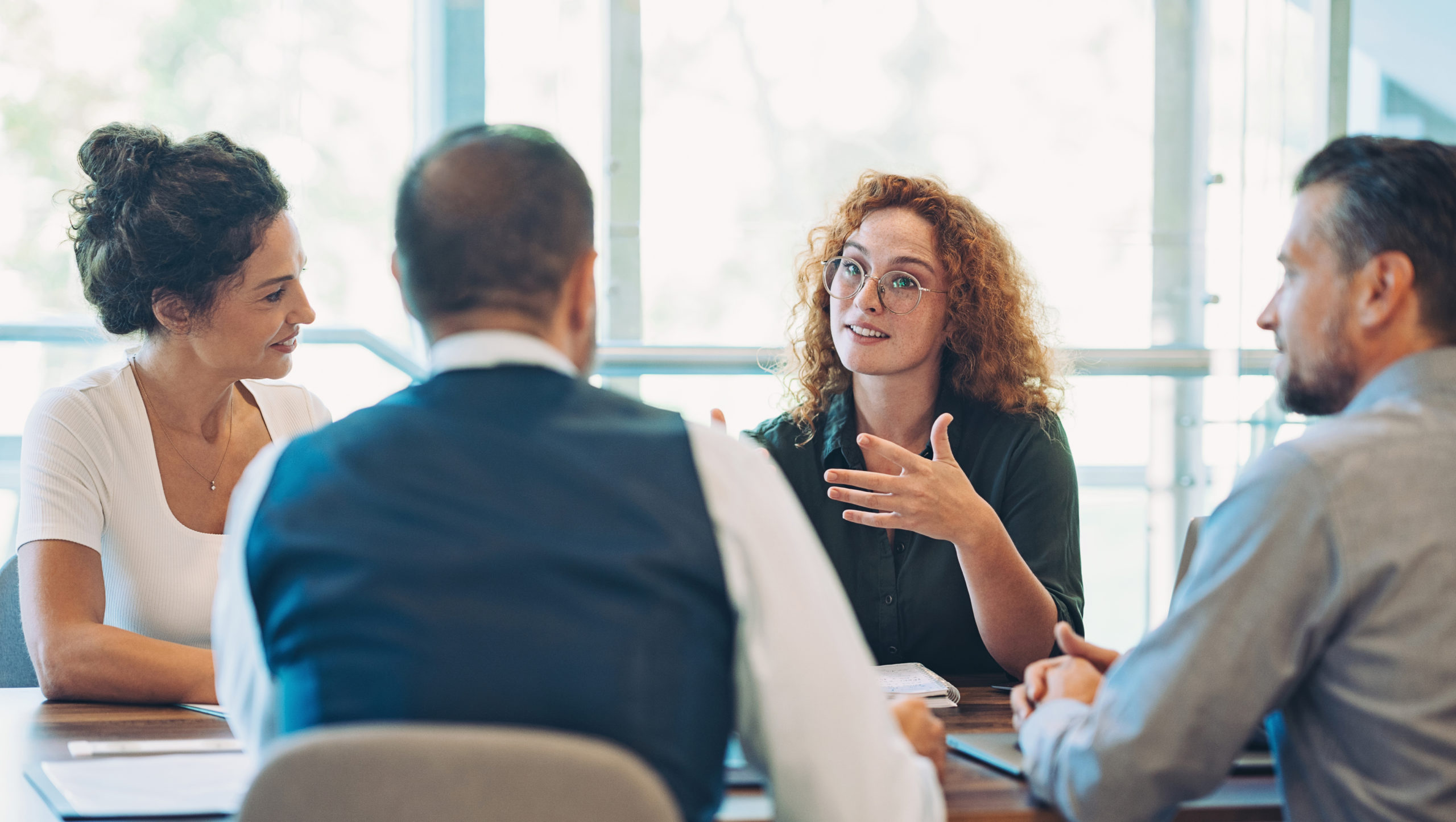
Advantages and opportunities
Adaptive learning offers a number of advantages that have a positive impact on the learning process. By adapting learning paths to the needs and level of knowledge of the individual, learners can be supported individually, which leads to more effective and sustainable learning outcomes. The adaptive approach also increases motivation and improves learning success, as learners are neither under- nor overchallenged. Self-directed and knowledge-based learning is strengthened because learners can influence their learning process. Companies benefit from more flexible training programmes that enable employees to develop their skills in a targeted manner without time-consuming repetition.
Another advantage is the possibility of using adaptive assessments. These dynamically adjust the difficulty level of exam questions. Based on a more precise diagnosis of the learner’s knowledge and needs, the learning material can be individually adapted. This makes the content more relevant and enables more efficient and targeted knowledge transfer. In addition, many learners perceive personalisation as a sign of appreciation, which increases their motivation. At the same time, detailed reporting enables an in-depth analysis of individual and collective strengths and weaknesses. This allows the learning content to be adapted based on data in order to address deficits in a targeted manner and continuously optimise the learning experience.
Challenges and restrictions
Despite the many advantages, there are also challenges. The development of adaptive learning content is complex and requires high resources in production and quality assurance. In addition, not all learning content is suitable for adaptive structures. Creative or collaborative learning methods, such as complex group projects or open forms of learning, are difficult to integrate into adaptive systems. Data protection and ethical issues should also not be underestimated, as adaptive systems have to analyse extensive personal data in order to guarantee their functionality.
One critical aspect is the black box problem with AI-controlled systems. If decision-making processes for learning recommendations are not transparent, this can undermine trust in adaptive learning systems. Clear documentation of the algorithms and decision-making logic is therefore essential. This detailed analysis of learning behaviour harbours a potential data protection risk. Particularly problematic is the possible creation of profiles in which learners are analysed over the long term. An ethically responsible design is essential in order to avoid misuse and discrimination.
future prospects
Digital education is developing rapidly and adaptive learning plays a key role in this. While personalised learning paths are already possible today, the future will be characterised by even more intelligent systems. The ability to optimise learning content in real time based on individual progress and preferences will become even more sophisticated.
One key aspect will be the integration of artificial intelligence and data-driven decision-making processes. AI can not only customise learning content, but also predict future learning needs and provide targeted support. At the same time, learning habits are changing, particularly among Generation Z and subsequent cohorts. The combination of adaptive learning formats and interactive, socially characterised learning environments will permanently change the educational landscape.
Interaction with AI
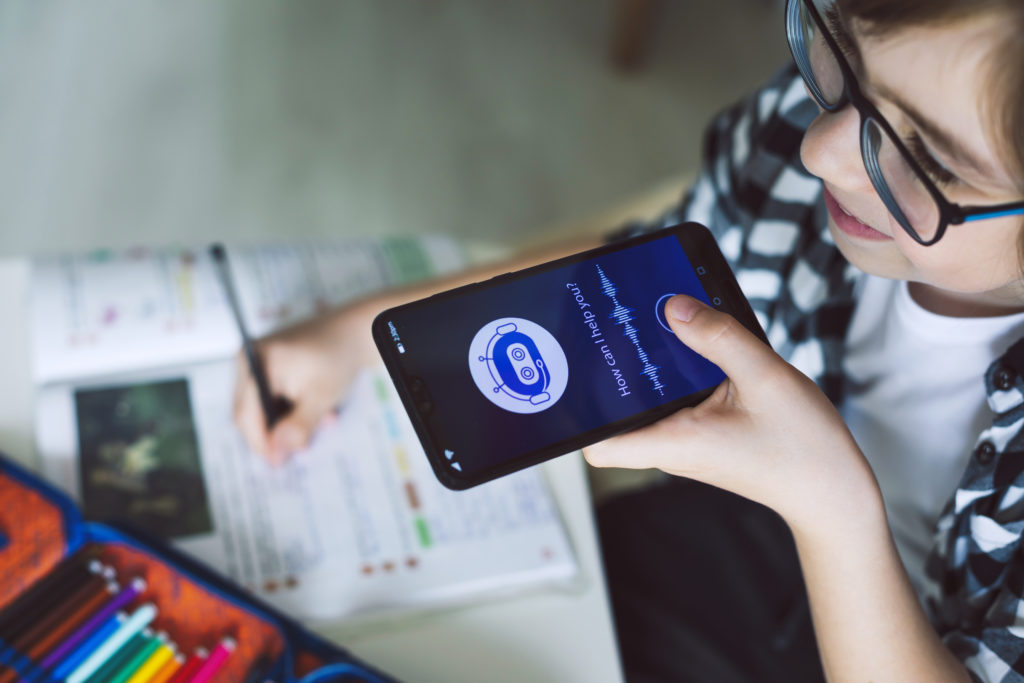
Artificial intelligence will have a significant impact on the development of adaptive learning systems. Machine learning and natural language processing (NLP) will enable adaptive systems to create even more precise learning profiles and generate customised content in the future. One promising development is the automated creation of learning materials based on AI-generated analyses that adapt to the needs of learners in real time.
A central aspect is deep learning, which makes it possible to analyse learning patterns over a longer period of time and make predictions about individual learning needs. This means that adaptive systems can not only react to current knowledge gaps, but also anticipate future difficulties and proactively suggest countermeasures. In addition, AI-supported speech recognition improves interaction with learning systems by using natural language processing to optimise questions and answers in real time. This leads to a more intensive and personalised learning experience.
Another promising development is the use of AI-supported tutors that provide personalised feedback and support learners in solving problems. These virtual assistants can be integrated both text-based and via voice or chatbots to enable interactive and in-depth learning experiences. At the same time, ethical issues of data protection and algorithmic transparency must be considered to ensure responsible use of the technology.
Learning behaviour of the young generation
The young generation is growing up in a digital world in which social media and interactive content play a central role. They therefore expect learning formats that focus on short, interactive and customisable content. Microlearning units, which can be consumed in just a few minutes, strike a chord with this generation and promote the continuous absorption of knowledge without overtaxing them. Adaptive learning can be targeted here by dividing learning content into small, dynamic sections and playing them out in a personalised way.
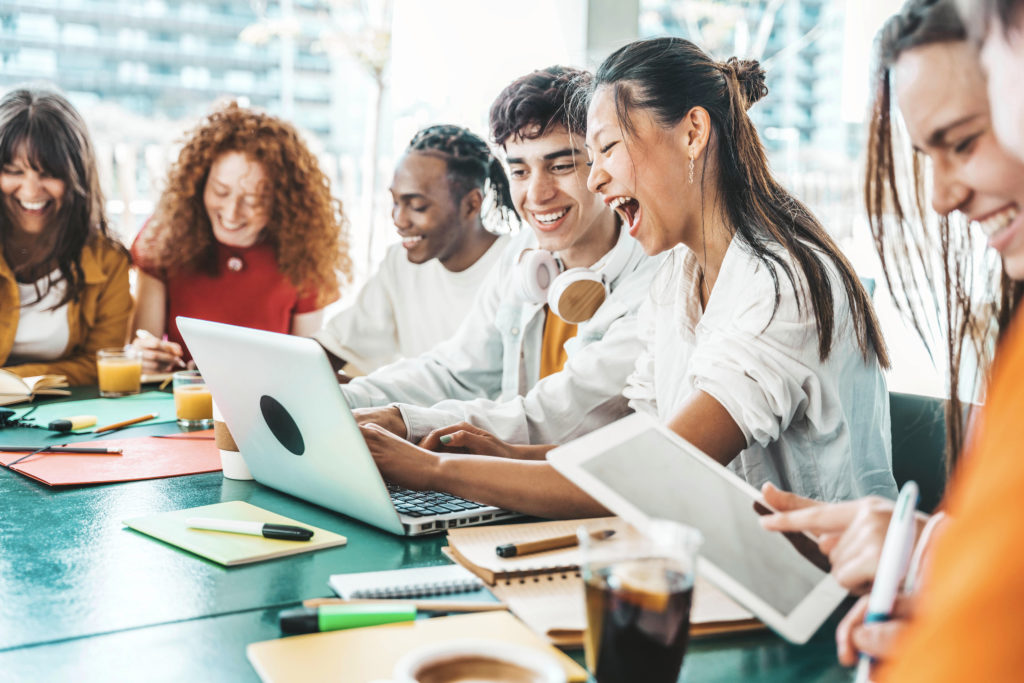
A decisive factor is gamification, which integrates playful elements such as points, rankings and rewards into the learning process. Studies show that playful mechanisms increase the motivation and commitment of learners. Formats that are based on social media platforms and classic game formats, such as interactive stories, communities, challenges or quiz formats that encourage competition and participation, are particularly successful.
Social learning, which promotes dialogue with other learners, is also increasingly in demand. AI-supported systems can suggest peer learning groups based on similar interests and learning progress. Live sessions with experts or interactive community functions are also playing an increasingly important role in supporting the social learning habits of Generation Z in the best possible way. Combining adaptive learning with these interactive, social and playful formats will be crucial to creating sustainable and effective learning processes for the learners of tomorrow.
Discover adaptability and promote knowledge in a targeted manner
With EVOLUTION³, you can create learning programmes that automatically adapt to the needs of your participants. Discover how you can use dynamic content and intelligent analyses to make your course a success. Get started now and set new standards in digital learning with EVOLUTION³!
How do we implement adaptivity in EVOLUTION³?
Our authoring platform EVOLUTION³ and our authoring tools EVOLUTION, FAST-TRAIN and FIRST-TRAIN offer a wide range of options for designing adaptive learning programmes. We have deliberately chosen to integrate adaptivity directly into the courses to enable tailor-made and flexible adaptation to individual learning needs. This allows us to use our own logic to control adaptivity and ensure that only data relevant to the decision at hand is collected.
Our learning programmes offer three main levels of adaptivity. Depending on the learner’s level of knowledge or answers, certain parts of the content (chapters, pages, sections or individual elements) are unlocked or skipped. EVOLUTION³ thus enables detailed control of the content structure and sequencing, so that the learning path adapts to the learner’s answers in real time. Another option is to conduct a knowledge test at the beginning of the course, which is used to tailor the subsequent content to the individual learner. This intelligent interplay of adaptivity ensures targeted, efficient and user-oriented knowledge transfer that maximises both relevance and learning success.
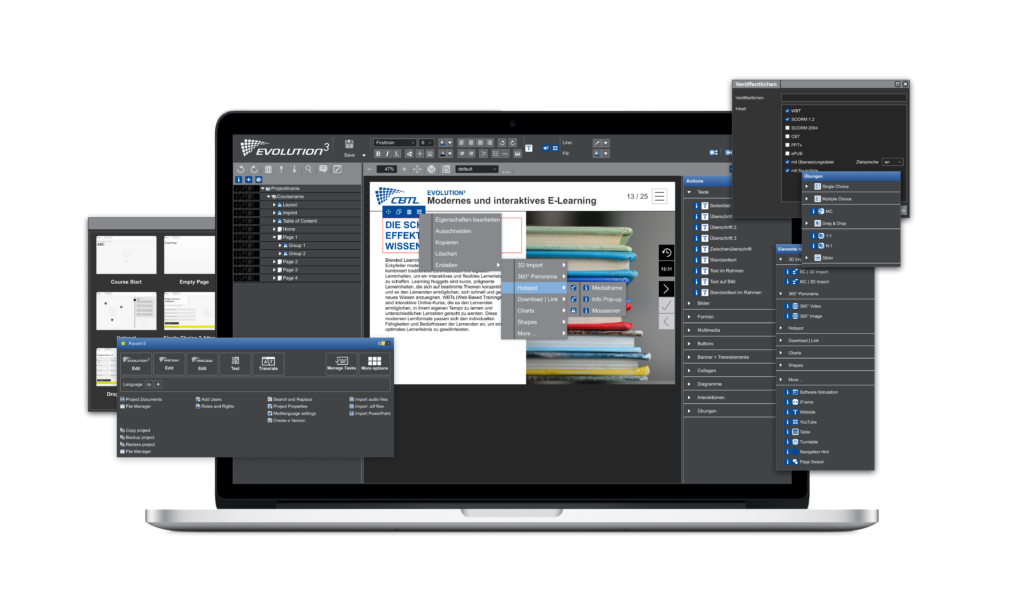
Adaptive chapter structure
- More options for adaptive learning at chapter or section level.
- Diagnostics through an initial test, followed by skipping of content based on the results.
- Depending on the test result or selection, different learning paths are taken in the module.
Adaptive page structure
- Definition of a sequence or random selection of questions.
- Selection of further questions based on user answers.
- Selection of a varying number of questions (e.g. in the final test) per content area based on performance in the corresponding area.
Adaptability on the page
- Unlock or skip certain sections based on user responses.
- By the way, showing more content for certain answers also applies to our feedback groups.
- Assessment of prior knowledge per chapter for different learning paths, supplemented by a final test.